How to Calculate Dispersion: A Clear Guide
Dispersion is an important concept in statistics that measures the spread of a set of data. It provides information about how the data is distributed around the central tendency. Dispersion is widely used in various fields, including finance, economics, and social sciences, to analyze and interpret data. In this article, we will explore the topic of dispersion and learn how to calculate various measures of dispersion.
Measures of dispersion are used to quantify the amount of variability or spread in a set of data. There are several measures of dispersion, including range, variance, standard deviation, and mean deviation. Each measure has its own advantages and disadvantages, and the choice of measure depends on the nature of the data and the purpose of the analysis. In general, a larger value of dispersion indicates that the data is more spread out, while a smaller value indicates that the data is more clustered around the central tendency.
Calculating dispersion requires some basic knowledge of statistics and mathematical concepts. However, with a little practice, anyone can learn how to calculate dispersion. The ability to measure dispersion is a valuable skill that can help in making informed decisions based on data analysis. In the following sections, we will discuss the different measures of dispersion and provide step-by-step instructions on how to calculate them.
Understanding Dispersion
Definition of Dispersion
Dispersion is a statistical term that refers to the spread or variability of a set of data. It is a measure of how much the individual data points deviate from the central tendency or mean value of the data set. Dispersion is important in statistics because it provides a way to understand how much the data varies and how representative the mean value is of the entire data set.
There are several measures of dispersion, including range, variance, standard deviation, mean deviation, and quartile deviation. Range is the simplest measure of dispersion and is calculated by subtracting the minimum value from the maximum value in the data set. Variance and standard deviation are more complex measures that take into account the variability of each data point from the mean. Mean deviation and quartile deviation are measures that provide information about the variability of the data around the median and quartiles, respectively.
Importance in Statistics
Understanding dispersion is important in statistics because it helps to identify outliers, or data points that are significantly different from the rest of the data set. Outliers can have a significant impact on the mean value of the data set, and therefore it is important to understand how much the data varies in order to determine whether the mean value is a representative measure of the entire data set.
Dispersion is also important in hypothesis testing, where it is used to determine whether the differences between two groups are statistically significant. If the dispersion of the data sets is large, it may be more difficult to detect differences between the groups, whereas if the dispersion is small, even small differences between the groups may be statistically significant.
In summary, dispersion is a key concept in statistics that provides important information about the spread or variability of a data set. By understanding dispersion, statisticians can determine whether the mean value is a representative measure of the entire data set and can identify outliers that may have a significant impact on the analysis.
Types of Dispersion Measures
There are several types of dispersion measures used in statistics to determine how spread out a set of data is. The most commonly used measures of dispersion are:
Range
Range is the simplest measure of dispersion. It is defined as the difference between the largest and smallest values in the data set. Range is easy to calculate, but it is sensitive to outliers and does not provide information about the distribution of the rest of the data.
Interquartile Range
Interquartile range (IQR) is a more robust measure of dispersion that is less sensitive to outliers. IQR is the difference between the third quartile (Q3) and the first quartile (Q1) of the data set. Quartiles divide the data into four equal parts, with Q1 representing the 25th percentile and Q3 representing the 75th percentile. IQR provides information about the spread of the middle 50% of the data.
Variance
Variance is a measure of how much the data deviates from the mean. It is calculated by subtracting each data point from the mean, squaring the result, and then taking the average of all the squared differences. Variance is sensitive to outliers and can be difficult to interpret, but it is commonly used in statistical analysis.
Standard Deviation
Standard deviation is the square root of the variance. It is a widely used measure of dispersion that is less sensitive to outliers than variance. Standard deviation provides information about how spread out the data is relative to the mean. In a normal distribution, approximately 68% of the data falls within one standard deviation of the mean, and approximately 95% falls within two standard deviations.
Mean Absolute Deviation
Mean absolute deviation (MAD) is a measure of how much the data deviates from the mean on average. It is calculated by taking the absolute value of the difference between each data point and the mean, and then taking the average of all the absolute differences. MAD is less sensitive to outliers than variance and standard deviation, but it is less commonly used.
Overall, understanding the different types of dispersion measures is important for accurately interpreting and analyzing data.
Calculating Range
Formula for Range
Range is a measure of dispersion that indicates the difference between the highest and lowest values in a dataset. It is calculated by subtracting the lowest value from the highest value. The formula for range is as follows:
Range = Maximum Value - Minimum Value
Example Calculation
Suppose a teacher wants to calculate the range of test scores for a class of 30 students. The scores range from 60 to 95. To calculate the range, the teacher would subtract the lowest score (60) from the highest score (95).
Range = Maximum Value - Minimum Value
Range = 95 - 60
Range = 35
Therefore, the range of test scores for this class is 35.
Calculating the range is a simple and quick way to understand the spread of data in a dataset. However, it only considers the highest and lowest values and does not take into account the distribution of the data.
Calculating Interquartile Range
Determining Quartiles
To calculate the interquartile range (IQR), you first need to determine the quartiles. Quartiles are values that divide a dataset into four equal parts, where the first quartile (Q1) marks the 25th percentile, the second quartile (Q2) marks the 50th percentile (also known as the median), and the third quartile (Q3) marks the 75th percentile.
To determine the quartiles, you need to order the dataset from lowest to highest and then find the median. The median divides the dataset into two halves, and you can find the first and third quartiles by finding the medians of the lower and upper halves, respectively.
Interquartile Range Formula
Once you have determined the quartiles, you can calculate the interquartile range using the formula:
IQR = Q3 - Q1
where Q3 is the third quartile and Q1 is the first quartile.
The interquartile range is a measure of dispersion that describes the spread of the middle 50% of the dataset. It is a more robust measure than the range because it is less affected by extreme values or outliers.
To summarize, calculating the interquartile range involves determining the quartiles by ordering the dataset and finding the medians of the lower and upper halves, and then using the formula IQR = Q3 - Q1 to calculate the range.
Calculating Variance
Variance is a measure of how spread out a set of data is. It is used to describe the degree of variation or dispersion in a set of data. Variance is calculated by taking the average of the squared differences from the mean. The formula for variance is given as:
$$\sigma^2 = \frac\sum_i=1^n(x_i-\mu)^2n$$
where:
- $\sigma^2$ is the variance
- $x_i$ is the ith element of the dataset
- $\mu$ is the mean of the dataset
- $n$ is the number of elements in the dataset
Population Variance
Population variance is the measure of variance of the entire population. It is calculated using the formula given above, where the mean is the population mean.
Sample Variance
Sample variance is the measure of variance of a sample taken from the population. It is calculated using the formula given above, where the mean is the sample mean. However, the formula for sample variance has a slight modification. Instead of dividing by the number of elements in the dataset, it is divided by the number of elements minus one. The formula for sample variance is given as:
$$s^2 = \frac\sum_i=1^n(x_i-\barx)^2n-1$$
where:
- $s^2$ is the sample variance
- $x_i$ is the ith element of the dataset
- $\barx$ is the sample mean
- $n$ is the number of elements in the sample
It is important to note that sample variance is an unbiased estimator of population variance.
In conclusion, variance is a measure of dispersion that is used to describe the degree of variation in a set of data. It is calculated using the formula given above and can be used to calculate both population and sample variance.
Calculating Standard Deviation
Standard deviation is a measure of the spread of a dataset. It tells you how much the data deviates from the mean. It is calculated by finding the square root of the variance. Standard deviation is a commonly used statistical measure and is useful in many fields, including finance, science, and engineering.
Standard Deviation Formula
To calculate the standard deviation, you need to follow these steps:
- Find the mean of the data set.
- Subtract the mean from each data point and square the result.
- Find the sum of the squared differences.
- Divide the lump sum loan payoff calculator by the number of data points minus one.
- Take the square root of the result.
The formula for standard deviation is as follows:
Where:
- σ is the standard deviation.
- x is each data point.
- x̄ is the mean of the data set.
- N is the total number of data points.
Interpreting Standard Deviation
Once you have calculated the standard deviation, you can interpret it in the following ways:
- If the standard deviation is small, the data is tightly clustered around the mean.
- If the standard deviation is large, the data is more spread out.
- If the standard deviation is zero, all the data points are the same.
In summary, standard deviation is a measure of how much the data deviates from the mean. It is calculated by finding the square root of the variance. Once you have calculated the standard deviation, you can interpret it to understand how the data is distributed.
Calculating Mean Absolute Deviation
Mean Absolute Deviation (MAD) is a statistical measure that describes the average distance between each data point and the mean of the data set. It is a useful measure of dispersion that provides information about the spread of the data set. Calculating MAD involves four simple steps.
Mean Absolute Deviation Formula
The formula for calculating MAD is as follows:
MAD = Σ|xi - X̄| / n
Where:
- MAD is the mean absolute deviation
- Σ represents the sum of the absolute deviations
- xi represents each data point in the data set
- X̄ represents the mean of the data set
- n represents the number of data points in the data set
To calculate MAD, one must first find the mean of the data set, then subtract each data point from the mean, take the absolute value of the result, and add up all the absolute deviations. Finally, divide the sum of the absolute deviations by the number of data points in the data set.
Application of Mean Absolute Deviation
MAD has several applications in various fields such as finance, economics, and engineering. In finance, MAD is used to calculate the risk of an investment portfolio. A portfolio with a higher MAD is considered to be riskier than a portfolio with a lower MAD.
In economics, MAD is used to measure the dispersion of economic data such as income, prices, and production. A higher MAD indicates that the data is more spread out, while a lower MAD indicates that the data is more clustered around the mean.
In engineering, MAD is used to measure the accuracy of a measurement system. A higher MAD indicates that the measurement system is less accurate, while a lower MAD indicates that the measurement system is more accurate.
Overall, MAD is a useful measure of dispersion that provides valuable information about the spread of a data set. By calculating MAD, one can gain insights into the variability of the data and make informed decisions based on the results.
Comparing Measures of Dispersion
Measures of dispersion are used to determine the spread of data. There are several measures of dispersion, and each one has its own strengths and limitations. In this section, we will discuss the most commonly used measures of dispersion, when to use each measure, and the limitations and considerations of each measure.
When to Use Each Measure
Range: Range is the simplest measure of dispersion and is calculated by subtracting the minimum value from the maximum value in a dataset. It is best used when the data is not normally distributed and has outliers. However, it does not take into account the distribution of the data, and it can be affected by outliers.
Mean Deviation: Mean deviation is calculated by finding the average of the absolute deviations from the mean. It is best used when the data is not normally distributed and has outliers. However, it is less commonly used than other measures of dispersion.
Variance: Variance is calculated by finding the average of the squared deviations from the mean. It is best used when the data is normally distributed and has no outliers. It is commonly used in statistical analysis and is the basis for calculating standard deviation.
Standard Deviation: Standard deviation is calculated by finding the square root of the variance. It is best used when the data is normally distributed and has no outliers. It is commonly used in statistical analysis and is a more robust measure of dispersion than variance.
Quartile Deviation: Quartile deviation is calculated by finding the difference between the 75th percentile and the 25th percentile. It is best used when the data is not normally distributed and has outliers. It is less sensitive to outliers than range.
Limitations and Considerations
Range: Range is affected by outliers, and it does not take into account the distribution of the data. It is also less robust than other measures of dispersion.
Mean Deviation: Mean deviation is less commonly used than other measures of dispersion, and it is less robust than other measures.
Variance: Variance is affected by outliers, and it is not as intuitive as other measures of dispersion. It is also sensitive to the units of measurement.
Standard Deviation: Standard deviation is affected by outliers, and it is sensitive to the units of measurement. However, it is a more robust measure of dispersion than variance.
Quartile Deviation: Quartile deviation is less sensitive to outliers than range, but it does not take into account the distribution of the data. It is also less commonly used than other measures of dispersion.
In conclusion, there are several measures of dispersion, and each one has its own strengths and limitations. The choice of measure depends on the distribution of the data and the presence of outliers. It is important to consider the limitations and considerations of each measure when choosing the appropriate measure of dispersion.
Frequently Asked Questions
What steps are involved in calculating dispersion in Excel?
To calculate dispersion in Excel, you need to have a data set ready. Once you have your data set, you can calculate the range, variance, and standard deviation using the appropriate formulas and functions in Excel. The steps involved in calculating dispersion in Excel are straightforward and can be easily accomplished by following a few simple steps.
What methods are used to calculate dispersion in research studies?
There are several methods used to calculate dispersion in research studies, including range, variance, standard deviation, mean deviation, and quartile deviation. The choice of method will depend on the nature of the data and the research question being addressed.
Can you provide an example of how to measure dispersion?
To measure dispersion, you can use the range, variance, or standard deviation of a data set. For example, if you have a data set of test scores for a class of students, you can calculate the range by subtracting the lowest score from the highest score. Alternatively, you can calculate the variance by finding the average of the squared differences between each score and the mean score, or you can calculate the standard deviation by taking the square root of the variance.
How do you determine the dispersion coefficient in a data set?
The dispersion coefficient is a measure of the degree of variation or spread in a data set. It can be calculated using the formula: dispersion coefficient = (standard deviation / mean) x 100%. The dispersion coefficient provides a useful way to compare the degree of variation in different data sets.
What is the process for calculating relative dispersion?
Relative dispersion is a measure of dispersion that takes into account the size of the data set. It is calculated using the formula: relative dispersion = (standard deviation / mean) x 100% x (1 / square root of n), where n is the size of the data set. The relative dispersion provides a way to compare the degree of variation in data sets of different sizes.
What constitutes an absolute measure of dispersion?
An absolute measure of dispersion is a measure of variation that is not affected by changes in the location or scale of the data. Examples of absolute measures of dispersion include range, variance, standard deviation, mean deviation, and quartile deviation. These measures provide a way to describe the degree of variation in a data set without being influenced by the specific values of the data.
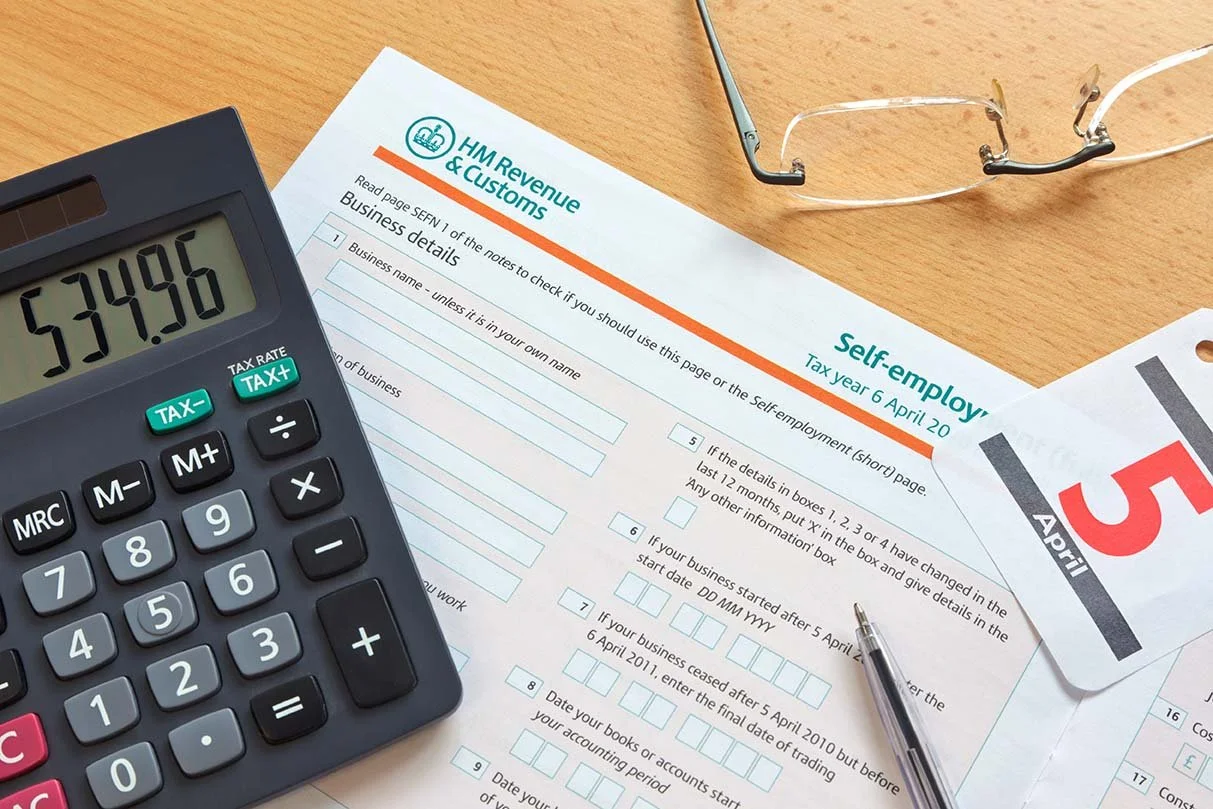