How to Calculate Spearman Rank Correlation: A Clear Guide
Spearman rank correlation is a statistical method used to measure the strength and direction of the relationship between two variables. It is a non-parametric method, which means that it does not assume any specific distribution for the data. Instead, it relies on the ranks of the data to calculate the correlation coefficient. This makes it a useful tool when dealing with data that is not normally distributed or when the relationship between the variables is not linear.
To calculate Spearman rank correlation, one needs to first assign ranks to the data, then calculate the difference between the ranks for each pair of observations, and finally compute the correlation coefficient using a formula. While the process may seem complicated at first, it can be broken down into simple steps that are easy to follow.
In this article, we will provide a step-by-step guide on how to calculate Spearman rank correlation. We will explain the underlying concepts and assumptions of the method, and provide examples to help readers understand how to apply it in practice. Whether you are a student, researcher, or data analyst, this article will equip you with the knowledge and skills needed to use Spearman rank correlation effectively in your work.
Understanding Spearman Rank Correlation
Spearman rank correlation is a statistical method used to measure the strength and direction of a relationship between two variables. It is a non-parametric test, which means it does not assume a normal distribution of the data. Instead, it uses the rank order of the data to calculate the correlation coefficient.
The Spearman rank correlation coefficient, also known as Spearman's rho (ρ), ranges from -1 to 1. A value of -1 indicates a perfect negative correlation, meaning that as one variable increases, the other decreases. A value of 1 indicates a perfect positive correlation, meaning that as one variable increases, the other also increases. A value of 0 indicates no correlation between the variables.
To calculate Spearman rank correlation, the data must first be ranked. The ranks are assigned based on the order of the values in the data set. For example, the smallest value is assigned a rank of 1, the second smallest a rank of 2, and so on. If there are ties in the data, the average rank is assigned to each tied value.
Once the data is ranked, the difference between the ranks for each pair of values is calculated. These differences are then squared and added together. The Spearman rank correlation coefficient is then calculated by dividing the lump sum payment mortgage calculator of the squared differences by a constant value, which is based on the sample size.
Spearman rank correlation is useful when the data does not follow a linear relationship. It can be used to analyze data that has a curvilinear or monotonic relationship, as well as ordinal data. It is also less sensitive to outliers than other correlation methods.
Overall, understanding Spearman rank correlation is important for analyzing the relationship between two variables when the data does not meet the assumptions of other correlation methods. By ranking the data and calculating the correlation coefficient, researchers can gain insight into the strength and direction of the relationship between the variables.
Prerequisites for Calculation
Data Types Applicable
Before calculating the Spearman rank correlation coefficient, it is important to ensure that the data being analyzed is appropriate for this type of analysis. The Spearman rank correlation is used to measure the strength of the relationship between two variables that are measured on an ordinal scale. This means that the data should be ranked in order of magnitude, rather than measured on a continuous or categorical scale.
Assumptions of Spearman Rank Correlation
There are a few assumptions that must be met before calculating the Spearman rank correlation coefficient. First, the data must be independent and randomly sampled from the population. Second, there should be no outliers in the data, as these can skew the results. Finally, the relationship between the two variables being analyzed should be monotonic, meaning that as one variable increases, the other variable either increases or decreases.
It is important to note that the Spearman rank correlation coefficient is a non-parametric measure of correlation, which means that it does not require any assumptions about the underlying distribution of the data. This makes it a useful tool for analyzing data that may not meet the assumptions required for other types of correlation analysis, such as the Pearson correlation coefficient.
In summary, before calculating the Spearman rank correlation coefficient, it is important to ensure that the data being analyzed is appropriate for this type of analysis, and that the assumptions of independence, no outliers, and monotonicity are met.
Step-by-Step Calculation Process
Ranking the Data
The first step in calculating Spearman's Rank Correlation Coefficient is to rank the data. This involves assigning a rank to each value in the dataset. The rank is simply the position of the value in the dataset when it is sorted in ascending order. For example, if the dataset is 3, 1, 4, 2, the ranks would be 2, 1, 4, 3.
Calculating the Difference in Ranks
Once the data has been ranked, the next step is to calculate the difference in ranks for each pair of values. This is done by subtracting the rank of one value from the rank of the other value. For example, if the ranks of two values are 2 and 4, the difference in ranks would be 2 - 4 = -2.
Squaring the Rank Differences
After the differences in ranks have been calculated, the next step is to square each of the differences. This is done to ensure that all of the differences are positive and to give more weight to larger differences. For example, if the difference in ranks is -2, squaring it would give a result of 4.
Summing the Squared Differences
Finally, the squared differences are summed to give the final value of Spearman's Rank Correlation Coefficient. This value can range from -1 to 1, with values close to -1 indicating a strong negative correlation, values close to 1 indicating a strong positive correlation, and values close to 0 indicating no correlation.
By following these four steps, anyone can calculate Spearman's Rank Correlation Coefficient for a given dataset. It is important to note that while this method can be used for any size dataset, it can become quite time-consuming for large datasets. In such cases, it may be helpful to use a statistical software package that can perform the calculations automatically.
Interpreting the Results
Correlation Coefficient Scale
The Spearman rank correlation coefficient, also known as Spearman's rho (ρ), ranges from -1 to 1. A correlation coefficient of -1 indicates a perfect negative relationship between the two variables, while a coefficient of 1 indicates a perfect positive relationship. A coefficient of 0 indicates no relationship between the two variables.
Interpreting the strength of the correlation depends on the absolute value of the coefficient. A coefficient between 0.1 and 0.3 indicates a weak correlation, a coefficient between 0.3 and 0.5 indicates a moderate correlation, and a coefficient greater than 0.5 indicates a strong correlation.
Significance of the Correlation
The significance of the correlation coefficient can be determined by conducting a hypothesis test. The null hypothesis is that there is no correlation between the two variables, while the alternative hypothesis is that there is a correlation.
The p-value is used to determine whether to reject or fail to reject the null hypothesis. If the p-value is less than the significance level (typically 0.05), then the null hypothesis is rejected, and it can be concluded that there is a significant correlation between the two variables. If the p-value is greater than the significance level, then the null hypothesis is not rejected, and it can be concluded that there is no significant correlation between the two variables.
It is important to note that a significant correlation does not necessarily imply causation. Correlation only indicates a relationship between two variables, but does not prove that one variable causes the other.
In summary, interpreting the results of a Spearman rank correlation involves examining the correlation coefficient scale and determining the significance of the correlation through hypothesis testing.
Use Cases for Spearman Rank Correlation
Spearman rank correlation is a non-parametric statistical method that measures the strength and direction of the monotonic relationship between two variables. It is a useful tool when working with ranked data, or when one or more extreme outliers are present.
One use case for Spearman rank correlation is in the field of education. For example, a dataset that contains the rank of a student's math exam score along with the rank of their science exam score in a class can be analyzed using Spearman rank correlation. This method can help determine if there is a significant correlation between a student's math and science scores.
Another use case for Spearman rank correlation is in the field of healthcare. For instance, researchers can use this method to determine if there is a correlation between a patient's age and their blood pressure. By ranking the patient's age and blood pressure values, researchers can evaluate if there is a monotonic relationship between the two variables.
Spearman rank correlation can also be used in the field of finance. For example, investors can use this method to determine if there is a correlation between the performance of two stocks. By ranking the performance values of the stocks, investors can determine if there is a monotonic relationship between the two stocks.
In summary, Spearman rank correlation is a useful tool for analyzing ranked data or when one or more extreme outliers are present. It can be used in various fields, including education, healthcare, and finance, to determine if there is a significant correlation between two variables.
Common Mistakes and Misinterpretations
Ignoring Outliers
One of the common mistakes when calculating Spearman's rank correlation is ignoring outliers. Outliers are data points that are significantly different from the other data points in the set. Ignoring outliers can lead to incorrect results and a misinterpretation of the correlation coefficient.
To avoid this mistake, it is important to identify and analyze outliers before calculating the correlation coefficient. One way to identify outliers is to use a scatter plot to visualize the data. Outliers will appear as data points that are far away from the other data points. Once identified, outliers can be removed from the data set or analyzed separately to determine their impact on the correlation coefficient.
Overreliance on Correlation Coefficient
Another common mistake when interpreting Spearman's rank correlation is overreliance on the correlation coefficient. It is important to remember that correlation does not imply causation. Just because two variables are highly correlated does not mean that one variable causes the other.
In addition, correlation coefficients can be misleading if the data set is small or if the correlation is weak. A weak correlation coefficient does not necessarily mean that there is no relationship between the variables. It could be due to a small sample size or the presence of outliers.
To avoid overreliance on the correlation coefficient, it is important to analyze the data set as a whole and consider other factors that may be influencing the relationship between the variables. It is also important to use other statistical tests to confirm the results obtained from Spearman's rank correlation.
Software and Tools for Calculation
Statistical Software Packages
There are several statistical software packages available that can calculate Spearman's rank correlation coefficient. Some popular options include:
- R: R is a free and open-source programming language and software environment for statistical computing and graphics. It has a built-in function called
cor.test
that can calculate Spearman's rank correlation coefficient. R is widely used in the academic and research communities. - SPSS: SPSS is a proprietary statistical software package that is commonly used in social science research. SPSS has a built-in function called
CORRELATIONS
that can calculate Spearman's rank correlation coefficient. - Stata: Stata is a proprietary statistical software package that is commonly used in academic research. Stata has a built-in function called
spearman
that can calculate Spearman's rank correlation coefficient.
Spreadsheet Applications
Many spreadsheet applications, such as Microsoft Excel and Google Sheets, also have built-in functions that can calculate Spearman's rank correlation coefficient. To calculate Spearman's rank correlation coefficient in Excel, users can use the CORREL
function. To calculate Spearman's rank correlation coefficient in Google Sheets, users can use the SPEARMAN
function.
It is important to note that while spreadsheet applications can be convenient for simple calculations, they may not be suitable for more complex statistical analyses. In these cases, it may be more appropriate to use a dedicated statistical software package.
Comparing Spearman Rank Correlation to Other Methods
Pearson Correlation
Pearson correlation is a parametric method that measures the linear relationship between two continuous variables. It assumes that the data is normally distributed and that the relationship between the variables is linear. Pearson correlation ranges from -1 to 1, where -1 indicates a perfect negative correlation, 0 indicates no correlation, and 1 indicates a perfect positive correlation.
Spearman rank correlation, on the other hand, is a non-parametric method that measures the monotonic relationship between two variables. It does not assume that the data is normally distributed and can be used for both continuous and ordinal data. Spearman correlation ranges from -1 to 1, where -1 indicates a perfect negative monotonic correlation, 0 indicates no monotonic correlation, and 1 indicates a perfect positive monotonic correlation.
Kendall Tau Correlation
Kendall Tau correlation is also a non-parametric method that measures the monotonic relationship between two variables. It is similar to Spearman correlation in that it does not assume that the data is normally distributed and can be used for both continuous and ordinal data. Kendall Tau correlation ranges from -1 to 1, where -1 indicates a perfect negative monotonic correlation, 0 indicates no monotonic correlation, and 1 indicates a perfect positive monotonic correlation.
One advantage of Kendall Tau correlation over Spearman correlation is that it is more robust to ties in the data. However, Spearman correlation is more commonly used in practice due to its ease of computation and interpretation.
In summary, Spearman rank correlation is a non-parametric method that measures the monotonic relationship between two variables and can be used for both continuous and ordinal data. It is a useful alternative to Pearson correlation when the data is not normally distributed or when the relationship between the variables is not linear. Kendall Tau correlation is another non-parametric method that is similar to Spearman correlation but is more robust to ties in the data.
Frequently Asked Questions
What is the process for handling tied ranks in Spearman rank correlation calculations?
Tied ranks are a common occurrence in data sets. When calculating Spearman's rank correlation coefficient, the tied ranks must be handled carefully. The most common method is to assign the average rank to all tied values. This will ensure that the rank order is preserved and that the correlation coefficient is calculated accurately.
How can Spearman's rank correlation coefficient be calculated using a standard calculator?
Spearman's rank correlation coefficient can be calculated using a standard calculator by following a few simple steps. First, calculate the rank for each value in the data set. Next, calculate the difference between the ranks for each pair of values. Then, square the differences and sum them up. Finally, use the formula to calculate the correlation coefficient.
What steps are involved in interpreting Spearman correlation results?
Interpreting Spearman correlation results involves examining the correlation coefficient, which ranges from -1 to 1. A coefficient of -1 indicates a perfect negative correlation, while a coefficient of 1 indicates a perfect positive correlation. A coefficient of 0 indicates no correlation. The sign of the coefficient indicates the direction of the correlation. The closer the coefficient is to -1 or 1, the stronger the correlation.
Can you provide a guide for calculating Spearman's rank correlation coefficient in Excel?
To calculate Spearman's rank correlation coefficient in Excel, first, rank the data set. Then, use the CORREL function to calculate the correlation coefficient. Make sure to specify the range of the ranked data set in the formula.
What are the necessary calculations for finding Rx and Ry in rank correlation?
To find Rx and Ry in rank correlation, first, calculate the rank for each value in the x and y data sets. Next, calculate the difference between the ranks for each pair of values. Then, square the differences and sum them up. Finally, use the formulas to calculate Rx and Ry.
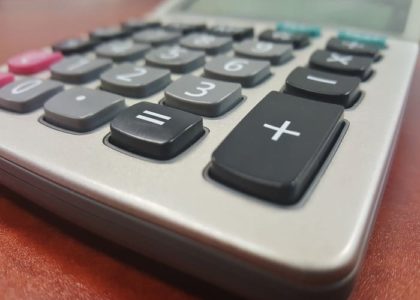
How is the Spearman rank correlation coefficient manually computed?
To manually compute the Spearman rank correlation coefficient, first, rank the data set. Then, calculate the difference between the ranks for each pair of values. Next, square the differences and sum them up. Finally, use the formula to calculate the correlation coefficient.